-
chevron_right
The XMPP Standards Foundation: The XMPP Newsletter March 2025
news.movim.eu / PlanetJabber · Saturday, 5 April - 00:00 · 7 minutes
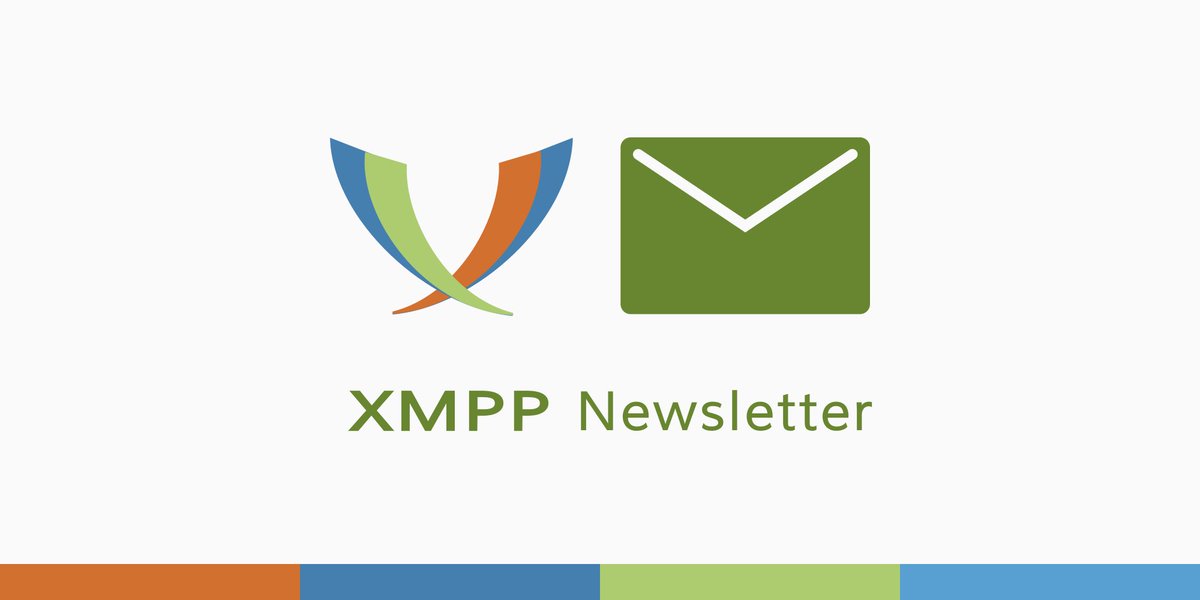
XMPP Newsletter Banner
Welcome to the XMPP Newsletter, great to have you here again! This issue covers the month of March 2025.
Like this newsletter, many projects and their efforts in the XMPP community are a result of people’s voluntary work. If you are happy with the services and software you may be using, please consider saying thanks or help these projects! Interested in supporting the Newsletter team? Read more at the bottom .
XSF Announcements
The XMPP Standards Foundation published an Open Letter to urge Meta to adopt XMPP for messaging interoperability. It’s time for real interoperability. Let’s make it happen.
XSF Membership
If you are interested in joining the XMPP Standards Foundation as a member, please apply until May 18th, 2025, 00:00 UTC! .
XSF Fiscal Hosting Projects
The XSF offers fiscal hosting for XMPP projects. Please apply via Open Collective . For more information, see the announcement blog post . Current projects you can support:
XMPP Events
- XMPP Track at FOSSY : Call for proposals is open until April 28th 2025! Once again this year, the soprani.ca project is pleased to announce its annual offer for funding to the potential attendees who may be struggling with financial limitations, especially to those who would like to give an XMPP related talk. Please, join us at discuss@conference.soprani.ca , and don’t hesitate to ask for more information.
- Berlin XMPP Meetup [DE / EN]: monthly meeting of XMPP enthusiasts in Berlin, every 2nd Wednesday of the month at 6pm local time.
- XMPP Italian happy hour [IT]: monthly Italian XMPP web meeting, every third Monday of the month at 7:00 PM local time (online event, with web meeting mode and live streaming).
- XMPP Sprint in Berlin : On Friday, 23rd, Saturday, 24th, and Sunday, 25th of May 2025.
Talks
- SASL-HT (The Hashed Token SASL Mechanism) was presented by Florian Schmaus at the 122nd meeting of the Internet Engineering Taskforce (IETF) . A recording is available on YouTube and the slides are available at the IETF 122 website . If adopted, this draft will become a “Request For Comments” (RFC) that will help standardize fast XMPP client reauthentication methods such as the one specified by XEP-0397 . The draft will be discussed further on the IETF Kitten working Group mailing list .
XMPP Articles
- Installer son serveur XMPP avec Prosody by Codimp has been updated to reflect the changes introduced by the latest Prosody 13.0.0 release [FR]
- Kaidan 0.12.0: User Interface Polishing and Account Migration Fixes by melvo for Kaidan IM .
- It’s time for real interoperability. Let’s make it happen! by guus for the Ignite Realtime News .
- Supporting XMPP Standard Foundation’s open letter to Meta for true interop by ProcessOne .
- Monocles , the project responsible for the XMPP Monocles chat Web and Android clients, started a crowdfunding campaign . The main goal is to create a platform where privacy, digital sovereignty and sustainability take center stage. Your privacy is non-negotiable. The funding period will span from 03.28.2025 to 06.30.2025.
XMPP Software News
XMPP Clients and Applications
- Gajim has released versions 2.0.1 , 2.0.2 , 2.0.3 and 2.0.4 with improvements and bug fixes. Head over to their News section for all the details.
- Kaidan has released versions 0.12.0 , 0.12.1 and 0.12.2 . These versions bring user interface polishing, account migration fixes, voice message and password change fixes, message removal and bubble fixes, along with more bugfixes. Check the changelog for each respective version here , here and here for all the details.
- Poezio has released versions 0.15.0 and 0.15.1 of their console XMPP client. Detailed information available on the release announcement .
- Profanity has released version 0.15.0 of their console based XMPP client. After more than a year and a half in the making, this release packages a large list of new features, improvements, bug fixes and more. You can find a detailed list of changes for this release on their changelog .
- prose-web-app has released versions 0.6.2 and 0.7.0 of its Web implementation of the Prose app.
XMPP Servers
- ProcessOne is pleased to announce the release of ejabberd 25.03 : a big release with new features and many improvements, as it comes three months and 180 commits after ejabberd 24.12.
- Prosody IM is pleased to announce the release of version 13.0.0 , the new major release of the Prosody XMPP server. This release brings a wide range of improvements that make Prosody more secure, performant, and easier to manage than ever before. Read about all the details on the release changelog . As always, detailed download and install instructions are available on the download page for your convenience.
XMPP Libraries & Tools
- go-xmpp versions 0.2.11 and 0.2.12 have been released.
- libstrophe version 0.14.0 has been released.
- peertube-plugin-livechat version 12.0.3 has been released.
- pelican-pubsub has been released. This is a plugin for the Pelican publishing system that implements support for Atom Over XMPP, through XMPP pubsub, enabling direct interaction (like publishing or adding comments) with Libervia and Movim users via XMPP.
- prose-core-client versions 0.1.101 and 0.1.102 have been released.
- python-nbxmpp versions 6.0.1 and 6.0.2 have been released.
- QXmpp versions 1.10.2 and 1.10.3 have been released.
- Slidge version 0.2.8 has been released.
- Slixmpp versions 1.9.1 and 1.10.0 have been released. You can read the respective release announcements here and here for all the details.
Extensions and specifications
The XMPP Standards Foundation develops extensions to XMPP in its XEP series in addition to XMPP RFCs . Developers and other standards experts from around the world collaborate on these extensions, developing new specifications for emerging practices, and refining existing ways of doing things. Proposed by anybody, the particularly successful ones end up as Final or Active - depending on their type - while others are carefully archived as Deferred. This life cycle is described in XEP-0001 , which contains the formal and canonical definitions for the types, states, and processes. Read more about the standards process . Communication around Standards and Extensions happens in the Standards Mailing List ( online archive ).
Proposed
The XEP development process starts by writing up an idea and submitting it to the XMPP Editor . Within two weeks, the Council decides whether to accept this proposal as an Experimental XEP.
- No XEPs proposed this month.
New
-
Version 0.1.0 of
XEP-0503
(Server-side spaces).
- Promoted to Experimental (XEP Editor: dg)
Deferred
If an experimental XEP is not updated for more than twelve months, it will be moved off Experimental to Deferred. If there is another update, it will put the XEP back onto Experimental.
- No XEPs deferred this month.
Updated
- No XEPs updated this month.
Last Call
Last calls are issued once everyone seems satisfied with the current XEP status. After the Council decides whether the XEP seems ready, the XMPP Editor issues a Last Call for comments. The feedback gathered during the Last Call can help improve the XEP before returning it to the Council for advancement to Stable.
- No Last Call this month.
Stable
- No XEPs moved to Stable this month.
Deprecated
- No XEPs deprecated this month.
Rejected
- No XEPs rejected this month.
Spread the news
Please share the news on other networks:
- Mastodon
- YouTube
- Lemmy instance (unofficial)
- Reddit (unofficial)
- XMPP Facebook page (unofficial)
Subscribe to the monthly XMPP newsletter
SubscribeAlso check out our RSS Feed !
Looking for job offers or want to hire a professional consultant for your XMPP project? Visit our XMPP job board .
Newsletter Contributors & Translations
This is a community effort, and we would like to thank translators for their contributions. Volunteers and more languages are welcome! Translations of the XMPP Newsletter will be released here (with some delay):
-
English (original):
xmpp.org
- General contributors: Adrien Bourmault (neox), Alexander “PapaTutuWawa”, Arne, Benson Muite, cal0pteryx, emus, Federico, Gonzalo Raúl Nemmi, Jonas Stein, Kris “poVoq”, Licaon_Kter, Ludovic Bocquet, Mario Sabatino, melvo, MSavoritias (fae,ve), nicola, Schimon Zachary, Simone Canaletti, singpolyma, XSF iTeam
-
French:
jabberfr.org
and
linuxfr.org
- Translators: Adrien Bourmault (neox), alkino, anubis, Arkem, Benoît Sibaud, mathieui, nyco, Pierre Jarillon, Ppjet6, Ysabeau
-
Italian:
notes.nicfab.eu
- Translators: nicola
-
Spanish:
xmpp.org
- Translators: Gonzalo Raúl Nemmi
-
German:
xmpp.org
- Translators: Millesimus
Help us to build the newsletter
This XMPP Newsletter is produced collaboratively by the XMPP community. Each month’s newsletter issue is drafted in this simple pad . At the end of each month, the pad’s content is merged into the XSF GitHub repository . We are always happy to welcome contributors. Do not hesitate to join the discussion in our Comm-Team group chat (MUC) and thereby help us sustain this as a community effort. You have a project and want to spread the news? Please consider sharing your news or events here, and promote it to a large audience.
Tasks we do on a regular basis:
- gathering news in the XMPP universe
- short summaries of news and events
- summary of the monthly communication on extensions (XEPs)
- review of the newsletter draft
- preparation of media images
- translations
- communication via media accounts
Unsubscribe from the XMPP Newsletter
To unsubscribe from this list, please log in first . If you have not previously logged in, you may need to set up an account with the appropriate email address.
License
This newsletter is published under CC BY-SA license .